Data is the new oil in more ways than one. For one thing, it’s an incredibly valuable resource, a crucial element of the modern economy. For another, it’s now the fuel that powers companies’ decision-making processes and business plans. People who can help companies harness it, such as data analysts and data scientists, are in high demand.
While both data analysts (you might also see them referred to as, for instance, “data scientists specializing in analytics”) and data scientists create value for companies by uncovering patterns in data, these roles involve different strengths and specializations.
If you’re thinking about a career in data, it’s important that you know how they differ in terms of skillsets, responsibilities, and salaries.
We’ll discuss these in turn, then look at what the Practicum bootcamp for each discipline involves. This should help you determine which one is a better fit for you.
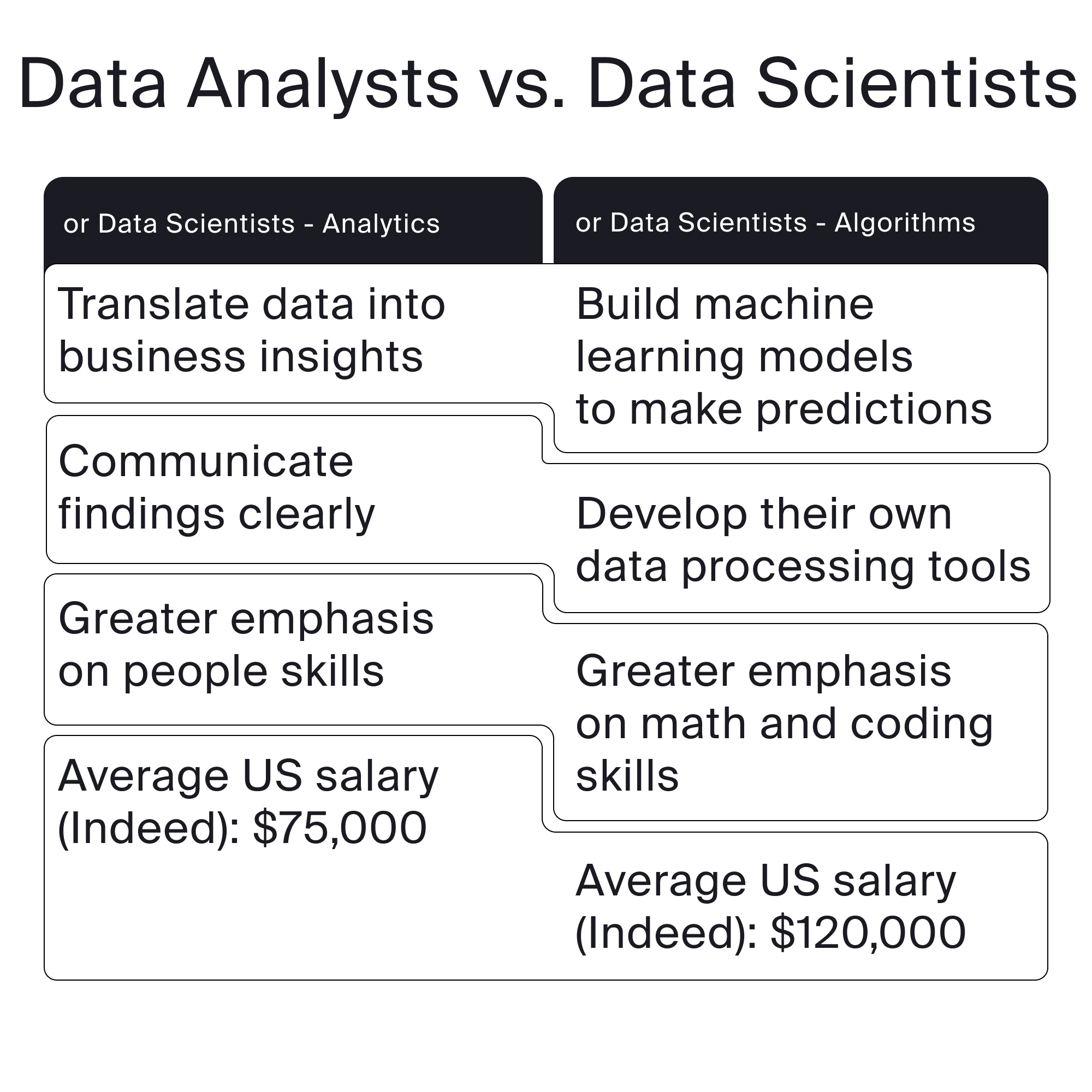
Hard skills
When it comes to hard skills for data analysts and data scientists, the main difference concerns the depth of technical knowledge they’re expected to have. This accounts in large part for the difference in salaries between the two jobs — more on that below.
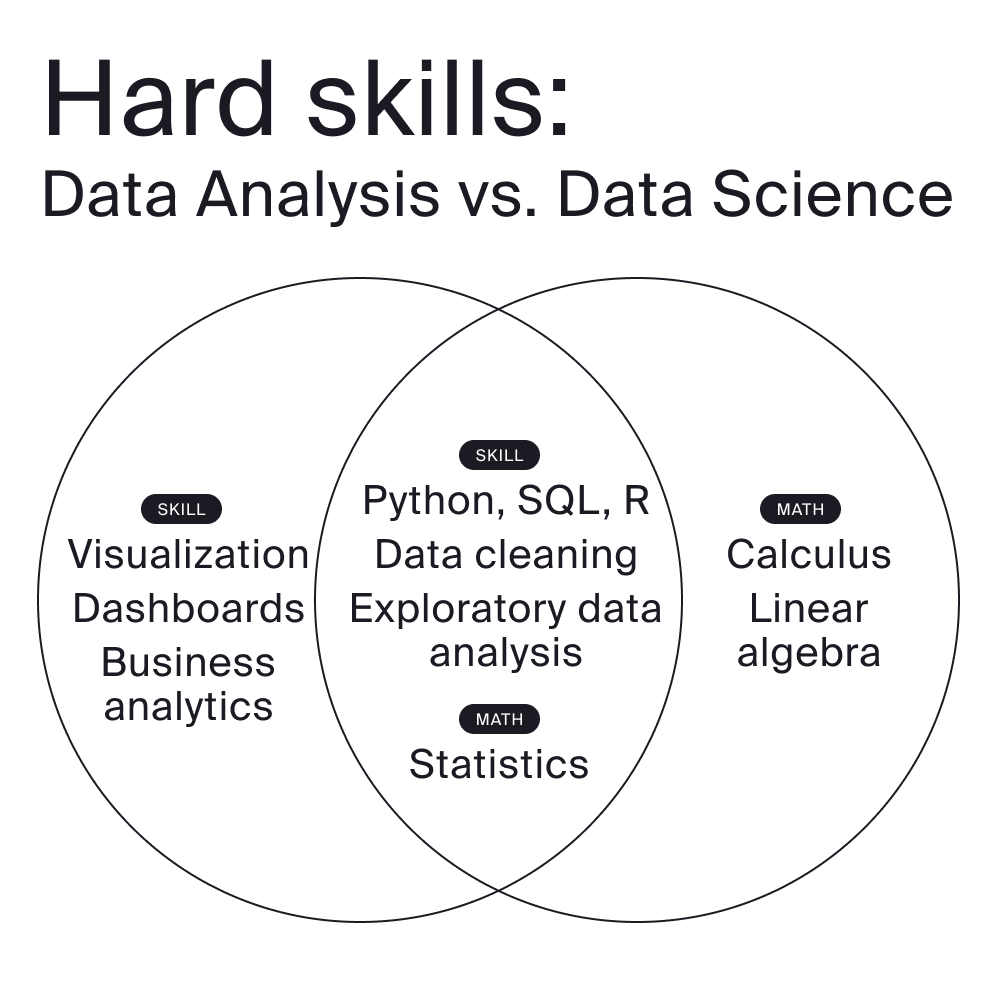
There’s some overlap in the technical skills required for data analyst and data scientist positions. The following are general requirements:
- Programming languages like Python and R (for reading, processing, and studying data)
- SQL (for working with databases)
- Probability and statistics (for testing statistical hypotheses [DA] and building ML models [DS])
So how do the roles differ?
Broadly speaking, data analysts identify and interpret patterns in datasets, formulate and test hypotheses, and draw conclusions that help employers make business decisions.
Thus, analysts need strong skills in the areas of business analytics, visualization (so that they can communicate their findings clearly), and dashboards (to automate the collection and visualization of data). They also need to understand the domain they’re working in; an analyst at a record label should know how the music industry functions, but that’s not true for an analyst at an insurance company.
While data analysts on the past and present, data scientists look more toward the future. Their forte is building models and generating algorithms in order to make predictions. Because machine learning is quite a technical field, data scientists are typically expected to have a deeper understanding of math and computer science than are analysts.
Although data scientists frequently use machine learning libraries such as PyTorch and LightGBM, it’s important for them to know the math underlying the tools they use — particularly statistics, calculus, and linear algebra — so that they can use those tools effectively.
Soft skills
People on the analytics side are often attached to business teams and need to be able to make their findings clear to non-specialists, meaning that communication skills are crucial.
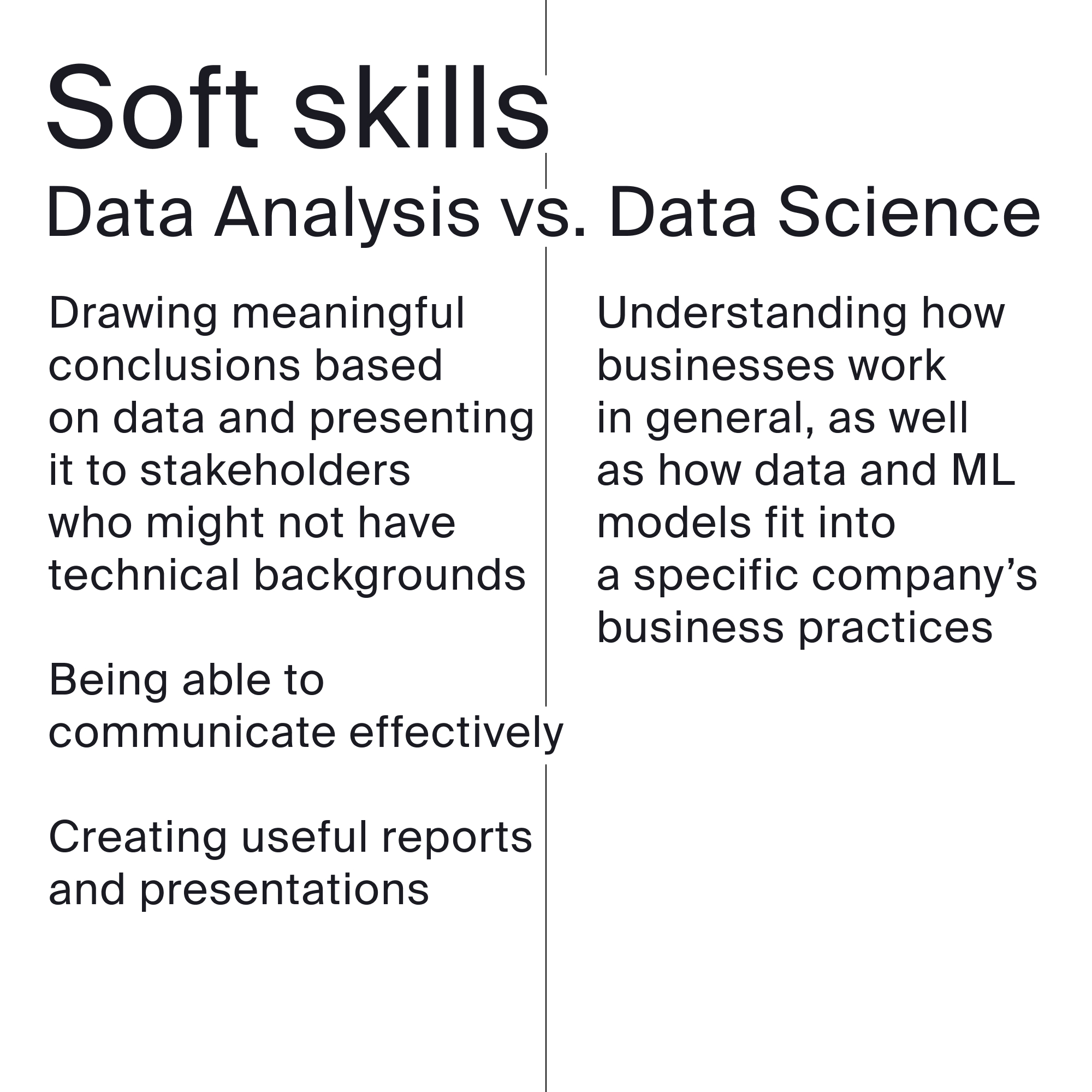
That’s not to imply that data scientists don’t need people skills at all. Since their work has real value and implications for businesses, it’s helpful for people in these roles to understand how businesses in general operate, as well as how data and machine learning models fit into a specific company’s processes. Still, for data scientists, deep technical expertise is the primary expectation.
Organizationally, data scientists tend to be a bit further removed from business teams, forming a separate group that works with different areas of a company as needed.
Responsibilities
Analysts are responsible for drawing conclusions based on data and communicating them to businesspeople and stakeholders who might not have technical expertise.

They act as go-betweens linking the technical and business sides of an organization and helping transform raw data into business insights. Often, their goal is to make data accessible to stakeholders and decision-makers (for instance, by creating automated dashboards that display and visualize the latest data, or by designing and fine-tuning reporting systems).
Data scientists, for their part, help organizations automate their decision-making processes. How likely is a certain customer to stop using the service next month, or to click on a particular advertisement? By answering questions like these, data scientists allow companies to root their strategies in data, rather than guesswork or intuition.
Salaries
Both disciplines are in demand. Data science came in third on LinkedIn’s Emerging Job report for the US this year, and the number of job openings for both data analyst and data scientist positions is expected to increase significantly over the next several years.
When it comes to salaries, however, there’s a big difference between the two specializations.
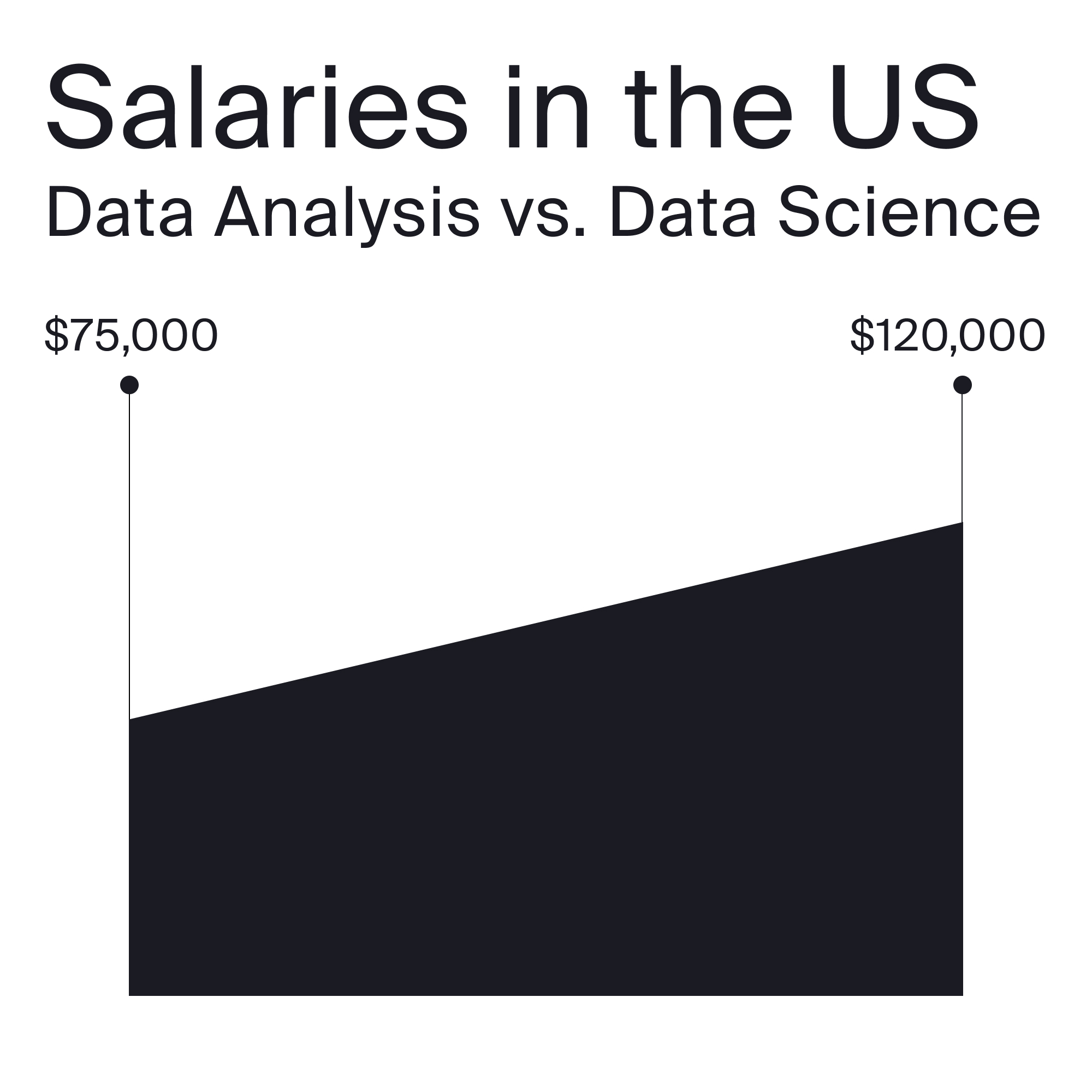
According to Indeed, the average base salary for data analysts in the U.S. is around $75,000 per year, which is higher than the median household income. However, the average base salary for a data scientist in the U.S. is significantly higher — currently over $120,000 per year. For senior data scientists, it’s over $150,000.
It’s worth noting that while for many, the word “data” is associated with the tech industry, that’s just one of the many places data analysts and data scientists can find jobs. All kinds of companies and organizations — banks, universities, government agencies — need professionals who can work with data
Which Practicum course is right for you?
Practicum’s Data Scientist and Data Analyst programs both begin with a free 20-hour intro course that teaches you some Python and gives you a sense of what it’s like to work with data. If you enjoy this course, you have the option of trying the full program.
Because both programs are designed to help you find work in the industry, they emphasize the use of important professional tools like the Jupyter development environment.
Both programs are practice-intensive, and you’ll graduate with a portfolio of projects to show to potential employers.
Whichever course you take, you’ll be expected to devote about 20 hours of work per week to it.
The Data Scientist program:
- Lasts eight months.
- Includes 16 projects.
Beyond the core courses it shares with Data Analyst, DS includes modules on linear algebra, machine learning, and more.
The Data Analyst program:
- Lasts six months.
- Includes 12 projects.
It includes courses on business analytics, creating visualizations and dashboards, and more.
Whether you choose the Data Analyst or Data Scientist track, you can take a career preparation course at no extra charge. Here you will polish your resume, put your portfolio in order, and complete mock interviews. Then, after you graduate from the program, Practicum will provide ongoing support as you search for a job.
Hopefully, you now have a better idea what sets these two similar-sounding specializations apart — and which one suits you better, personally and professionally. Becoming a data analyst or data scientist is challenging but doable, and a Practicum course can prepare a student for an entry-level job in either profession.
Find out more about Practicum’s Data Science and Data Analysis programs.
Which program sounds more appealing, and why? Can you think of any other differences between data analysts and data scientists?